Compare large language models vs generative AI
The Forrester Wave: Conversational AI For Customer Service 2024 Top Takeaways
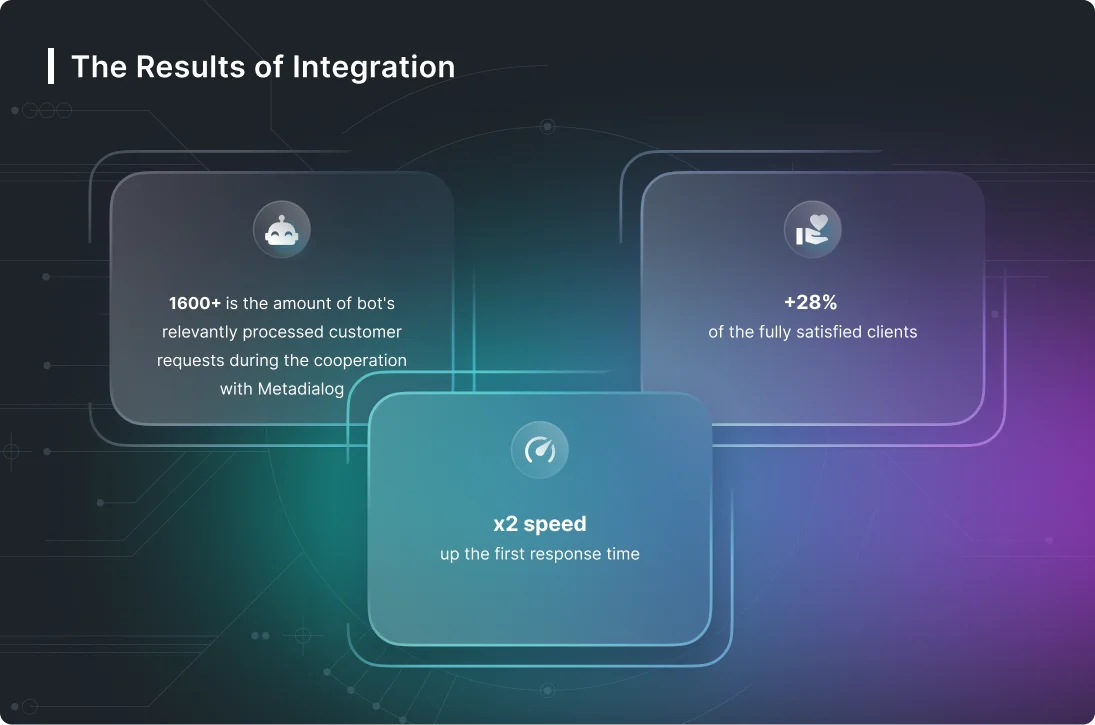
IBM watsonx Assistant can now give prospects, customers, and employees conversational answers based on an organization’s proprietary, or public facing content without human authors having to write a single line of text. Once watsonx Assistant is connected to a knowledge base for conversational search, it automatically pulls information from that source to inform its generated answers. When information changes or new information becomes available, teams can simply update the information in their knowledge base.
- Aside from their respective functions, there are also differences when it comes to how these technologies operate.
- However, prior research also shows that people with more severe symptoms showed a preference for human support37.
- This is really taking their expertise and being able to tune it so that they are more impactful, and then give this kind of insight and outcome-focused work and interfacing with data to more people.
- Today, we are excited to announce the beta release of Conversational Search in watsonx Assistant.
One possible explanation might be the variations in engagement levels, but due to the high heterogeneity across studies, we were unable to validate these assumptions. Future research is warranted, as a prior review suggests a curvilinear relationship between age and treatment effects59. Notably, we did not find a significant moderating effect of gender, consistent with earlier findings demonstrating that digital mental health interventions are similarly effective across genders60. In doing so, these providers have enabled customers to build bots using natural language alone, simulate conversations for testing, and adapt the tone of replies based on customer responses. Using conversational data analytics, businesses gain valuable insights into customer preferences, sentiments and pain points. They can use these insights to further refine products, tailor marketing campaigns and provide better customer support.
Differences between conversational AI and generative AI
These bots can also draw information from CRM systems and databases, examine previous conversation histories, and ensure every user receives a unique experience. Conversational analytics tools have become an essential component of the customer experience space in recent years. These solutions leverage natural language processing and understanding technologies, alongside AI and machine learning to assist businesses in unlocking valuable insights. Generative AI and conversational AI tools are beginning to work together in the customer experience landscape, empowering businesses to produce not only more valuable chatbots and virtual assistants, but also more engaged, productive teams. This webinar highlighted how a Generative AI-based conversation analytics foundation can provide a deeper understanding of 100% of all calls and conversations within very quick timeframes.
Capable of creatively simulating human conversation, through natural language processing and understanding, these tools can transform your company’s self-service strategy. Machine learning, especially deep learning techniques like transformers, allows conversational AI to improve over time. Training on more data and interactions allows the systems to expand their knowledge, better understand and remember context and engage in more human-like exchanges. Since Conversational AIis dependent on collecting data to answer user queries, it is also vulnerable to privacy and security breaches. Developing conversational AI apps with high privacy and security standards and monitoring systems will help to build trust among end users, ultimately increasing chatbot usage over time. Natural language processing is the current method of analyzing language with the help of machine learning used in conversational AI.
Usually, the generative AI will automatically save your conversations, ergo you can switch back and forth between a multitude of such conversations and not worry about them being inadvertently tossed asunder or otherwise lost. You probably know that when using a generative AI app such as the widely and wildly popular ChatGPT by AI maker OpenAI you do so via what is referred to as “conversations”. A conversation consists of you entering prompts and the generative AI generating responses to your prompts. The idea is that this is akin to having a conventional type of conversation with a human, namely that the accepted norm of how we converse with each other as humans consists of taking turns when expressing ourselves. One such early version solution has been recently and prominently announced and I will be walking you through the particulars of that pronouncement. For my ongoing and extensive coverage of the latest in AI and what’s happening, see my column analyses at the link here.
Raizor and Teneo.AI Join Forces to Deliver Generative AI and Conversational AI for Enterprise Contact Centers — Cision News
Raizor and Teneo.AI Join Forces to Deliver Generative AI and Conversational AI for Enterprise Contact Centers.
Posted: Wed, 08 Jan 2025 08:00:00 GMT [source]
This same analysis is to be repeated, namely each new conversation becoming a source conversation that begets more snippets. If you then have had say a dozen conversations, they are all construed ultimately as source conversations. When the next conversation is undertaken, consider it to be the thirteenth conversation (maybe an unlucky number!), and it now momentarily becomes the target conversation (inevitably another source conversation too). Let’s consider the overall process of when snippets would be first uncovered and how they would be later utilized.
Although LLMs are an important part of the generative AI landscape, they’re only one piece of a bigger picture. The Infosys Innovation Network (IIN) is a well-orchestrated partnership between select startups and Infosys to provide innovative services to our clients. The IIN program aims to create lighthouse wins for clients to experiment and implement art-of-the-possible. Infosys de-risks client adoption of technology products and solutions by carefully curating these startups, finding the right fit, and implementing early pilots.
Join The Conversation
There have been concerted efforts to consider that humans have an IQ (intelligence quotient), and an EQ (emotional quotient), and some suggest there is even a CQ or C-IQ (conversational related). AI researchers are typically trying to come up with a data structure that can be beneficially used to represent conversations, and likewise devise a type of specialized lingo or language to describe the nature of conversations. By doing so, the ability to develop AI that can parse and process conversations is going to be advanced. This approach entails being able to slice and dice a conversation, being accomplished at varying levels of granularity.
In Western cultures, at least, linguists often regard conversation as proceeding according to four principles or “maxims” set out in 1975 by British philosopher of language Paul Grice. The authors distinguish between formal linguistic competence (knowledge of rules and statistical patterns in language) and functional linguistic competence (how to use language in real-world situations). Earlier this year, a Hong Kong finance worker was tricked into paying US$25 million to scammers who had used deepfake technology to pretend to be the company’s chief financial officer in a video conference call. Thinking the images on screen were his colleagues, the financier authorised the multi-million dollar transfer to fraudsters posing as friends. Celeste Rodriguez Louro does not work for, consult, own shares in or receive funding from any company or organisation that would benefit from this article, and has disclosed no relevant affiliations beyond their academic appointment. Like most forms of AI, generative AI relies on access to large volumes of data, which needs to be protected for compliance purposes.
Customers are also increasingly expectant of an omnichannel customer experience. As such, businesses will seek to create a seamless transition between messaging apps, websites, social media, and phone lines so customers can pick up queries across multiple channels. Moreover, by leveraging generative AI, virtual agents may now have more human-like conversations, modulate tone, and personalize responses for each individual customer, across various languages. The unified release of IBM watsonx Orchestrate is now generally available, bringing conversational AI virtual assistants and business automation capabilities to simplify workflows and increase efficiency. One of the biggest benefits of generative AI in the contact center is its ability to support employees in rapidly automating tasks, without the need for complex coding and workflow building. Generative AI can complete tasks with nothing but natural language input from team members.
Orchestrate is personalized with the skills to support the work of your teams, using the tools they already use. With an expanding catalog of pre-built skills and capabilities to discover and build automations from third-party applications, RPA, workflow and decisions integrations, business users can coordinate common and complex tasks. From creating a job description or pulling a report in Salesforce to sourcing candidates and generating sales offers, all driven by intuitive natural language.
Data availability
Should it stick to the task, engage in trivia and small talk, or go “completely freestyle”? Previously, chatbot designers had to plug all the possible ways a customer could ask a question into their bot for each intent. The tool may then create such a Lexicon, which the airline can review, finetune to their flight plan, and embed into their bots. Some models – including those offered by Cognigy and Kore.ai – may even define the necessary API integration to complete the flow. They may then select use cases for the bot from this list and – with the click of a button – generate prospective bot flows across each intent (more on this below!). The developer can then enter a description to unlock more use cases, which they can add to the chatbot – as the screenshot below highlights.
As Conversational AI technologies evolve, contact center managers and AI owners will increasingly leverage sophisticated voice and chat analytics. As a result, generative AI advancements will accelerate conversational AI adoption rates in contact centers. Yet, Metrock also suggested that many vendors may face a new “existential risk” as the conversational AI market becomes increasingly crowded. “It’s going to be a wild year in conversational AI,” predicted Bradley Metrock – a prominent market thought leader – during a recent CX Today interview. “Lack of mature technology, adequate policies and procedures, training, and safeguards are creating a perfect storm for AI accidents far more dramatic than just hallucinations.
Predictive AI use cases
They also highlighted the Thomson Reuters acquisition of Materia, an AI assistant and platform for accounting and auditing professionals. Discover how to use AI-generated customer review highlights with these step-by-step instructions. Its use will gradually grow over time and, little by little, alter and transform human activities.

As you can see, the language models are at the bottom of the landscape because they form the fundamental building blocks of natural language processing (NLP) used for all the other functions. The sampling of language models shown here includes OpenAI’s GPT, Google’s LaMDA and BigScience’s BLOOM. ChatGPT is built on OpenAI’s GPT language model and provides a variety of functions, such as engaging in conversations, answering questions, generating written text, debugging code, conducting sentiment analysis, translating languages and much more. Google Cloud’s blast of new generative AI features for Vertex AI at the Cloud Next event went beyond models with the reveal of two new interactive tools, Vertex AI Search and Vertex AI Conversation.
Discover, create and deploy automations as skills
Text translation companies use AI to translate written texts from one language to another. It refers to AI technology that can create original content such as text, image, video, audio and code. Our landscape is focused on the area of text generative AI because that’s the predominant function of ChatGPT. Ensuring robust data protection measures and obtaining explicit user consent become critical aspects for responsible chatbot implementation. You can also click the “Analytics” button to summarize various statistics linked to agent requests and responses.
I’ll pick the example about having mentioned in a ChatGPT conversation that you have a toddler, and that the toddler loves jellyfish. We might want to give users of generative AI the capability to peruse the snippets and decide which ones they want to delete. Lots of tough computational choices need to be made about how to reuse conversational snippets.
In some sense, they don’t experience the limitation because they always start a conversation on a new topic and do not care whether any prior conversations with the generative AI are carried into the new discussion. Necessity answers that leveraging prior conversations is the sensible way to go. I dare suggest that if we were all moment-to-moment stuck with having to start each conversation brand new, the amount of time and effort to bring each other up to speed would be enormous.
Envision that you had started the new conversation about birthday planning and asked ChatGPT to provide gift ideas for your toddler. Assuming that the conversational sharing mode is active, once again the jellyfish reference might automatically come to the fore. I would like to point out that this same example could have gone somewhat awry. I am not trying to be a party pooper and only want to provide balance about what can take place with conversational interlacing.
This technology, which relies heavily on large language models trained on vast amounts of data to learn and predict the patterns of language, has become increasingly widespread since the launch of ChatGPT in 2022. Raizoris a cutting edge Artificial Intelligence delivery organisation that solves real-world business problems with practical application of Generative and Conversational AI solutions, centered on an effortless Customer Experience. With over 25 years in the CX industry, they provide consulting, implementation, integration and managed services to Enterprise businesses and BPO’s around the world. One major use case for generative AI in the contact center is the ability to automate repetitive tasks, improving workplace efficiency. Generative AI bots can transcribe and translate conversations like their conversational alternatives and even summarize discussions. A total of 14 distinct psychological outcomes were evaluated in the 35 studies.
As AI technology evolves, it is crucial to address the challenges of privacy, bias and misinformation. LLMs are trained on hundreds of billions more words than a typical human encounters by age 10 and display a sophisticated capability for language processing and conversational response generation. Let’s say a customer opens the bank’s assistant and asks what sort of welcome offer they would be eligible for if they apply for the Platinum Card. Watsonx Assistant leverages its transformer model to examine the user’s message and route to a pre-built conversation flow that can handle this topic. The assistant can seamlessly and naturally extract the relevant information from the user’s messages to gather the necessary details, call the appropriate backend service, and return the welcome offer details back to the user.
We’ve examined some of the top conversational AI solutions in the market today, to bring you this map of the best vendors in the industry. Most generative AI models lack explainability, as it’s often difficult or impossible to understand the decision-making processes behind their results. Conversely, predictive AI estimates are more explainable because they’re grounded on numbers and statistics. But interpreting these estimates still depends on human judgment, and an incorrect interpretation might lead to a wrong course of action.
All these qualities enable better chat experiences, as many of the following use cases exemplify. And they are more the orchestrator and the conductor of the conversation where a lot of those lower level and rote tasks are being offloaded to their co-pilot, which is a collaborator in this instance. But the co-pilot can even in a moment explain where a very operational task can happen and take the lead or something more empathetic needs to be said in the moment.
With the report claiming that “GenAI will save the beleaguered chatbot,” Forrester argues that the technology’s inherently natural conversations and significantly reduced deployment times are a self-service game-changer. However, when it comes to more diverse tasks that require a deeper understanding of context, NLP models lack the capacity to generate new content. Because NLP models are focused on language rules, ambiguity can lead to misinterpretations. NLP is a branch of AI that is used to help bots understand human intentions and meanings based on grammar, keywords and sentence structure.
Generative AI & Conversational Analytics for Customer Experience
Like conversational AI, generative AI tools can have a huge impact on customer service. They can understand the input shared by customers in real time and use their knowledge and data to help agents deliver more personalized, intuitive experiences. The rapid advancement of artificial intelligence (AI) and machine learning (ML) technologies is pushing the boundaries of what can be achieved in marketing, customer experience and personalization. One important development is the ongoing evolution of generative AI (gen AI), which is bringing open-source platforms to the forefront of sales. As the digital-first business landscape grows increasingly complex and fast-paced, these technologies are becoming indispensable tools. Virtual assistant software responds to human language and helps the user with a variety of tasks and queries.
LLMs are beneficial for businesses looking to automate processes that require human language. Because of their in-depth training and ability to mimic human behavior, LLM-powered CX systems can do more than simply respond to queries based on preset options. In contrast to less sophisticated systems, LLMs can actively generate highly personalized responses and solutions to a customer’s request. LLMs are a type of AI model that are trained to understand, generate and manipulate human language. LLMs, such as GPT, use massive amounts of data to learn how to predict and create language, which can then be used to power applications such as chatbots. With solutions for digital workplace management, employee engagement, and cognitive contact center experiences, Eva addresses various enterprise use cases.
Additionally, sometimes chatbots are not programmed to answer the broad range of user inquiries. When that happens, it’ll be important to provide an alternative channel of communication to tackle these more complex queries, as it’ll be frustrating for the end user if a wrong or incomplete answer is provided. In these cases, customers should be given the opportunity to connect with a human representative of the company. The meta-analyses were conducted using R software (version 3.6.2) and the metafor package. Data were extracted from RCTs to calculate pooled effect sizes of Hedges’ g, with corresponding 95% confidence intervals and P-values. Hedges’ g of 0.2 indicated a small effect, 0.5 a moderate effect and 0.8 a large effect66.
Robisco, on the other hand, is optimistic about this and believes that it will only remove the most repetitive tasks, leaving the most creative, important and value-added part to humans. Given generative AI’s potential and upward progress, it raises many questions. One of the most controversial and feared is that it may take away jobs, if it is not already doing so — at least the most repetitive and automatable.
- The sampling of language models shown here includes OpenAI’s GPT, Google’s LaMDA and BigScience’s BLOOM.
- The company’s platform uses the latest large language models, fine-tuned with billions of customer conversations.
- So they really have to understand what they’re looking for as a goal first before they can make sure whatever they purchase or build or partner with is a success.
- Implemented in tandem with our existing platform, we seek to utilize LLMs to build more efficient systems that don’t compromise accuracy and security but drive seamless customer experiences.
- The resulting text generative and conversational AI Landscape is shown below and consists of ten functional categories with a sampling of representative companies for each category.
With the right features enabled, you can use Dialogflow CX and the Gen App Builder console to rapidly create, configure, and deploy your virtual agent. Meanwhile, we see voice as the next evolutionary step for generative AI and the next phase for conversational AI to fully enter the mainstream. Advances in the conversational AI space have this technology, along with generative AI, uniquely positioned to be the battle axe needed to win with CX Led Growth. To do so, they must discover customer needs, design solutions to meet them, and deliver business impacts with cross-functional reporting that empowers continual change and improvement. Indeed, multi-modality is driving the need for a holistic approach to conversational AI, as each channel offers unique benefits and limitations.

They are based on the older stilted ways of natural language processing (NLP), which has frustrated people to no end. Most people end up crimping their fluency to get those AI apps to do what is requested. In line with the vast amount of models and tools under its umbrella, generative AI has many use cases.
A recent study from Zendesk found that 70% of CX leaders plan to integrate AI into many customer touchpoints within the next two years, while over half of respondents expressed their desire to increase AI investments by 2025. In turn, customer expectations have evolved to reflect these significant technological advancements, with an increased focus on self-service options and more sophisticated bots. With an easy-to-use platform, Google empowers teams to develop custom agents in a few clicks, with Vertex AI Search and Conversation, within the Dialogflow UI. There are visual flow builders, support for omnichannel implementation, and state-based data models to access. Google also offers a range of technical and training resources to AI beginners.